Héctor Climente-González
Lead Scientist at Novo Nordisk. London, United Kingdom.
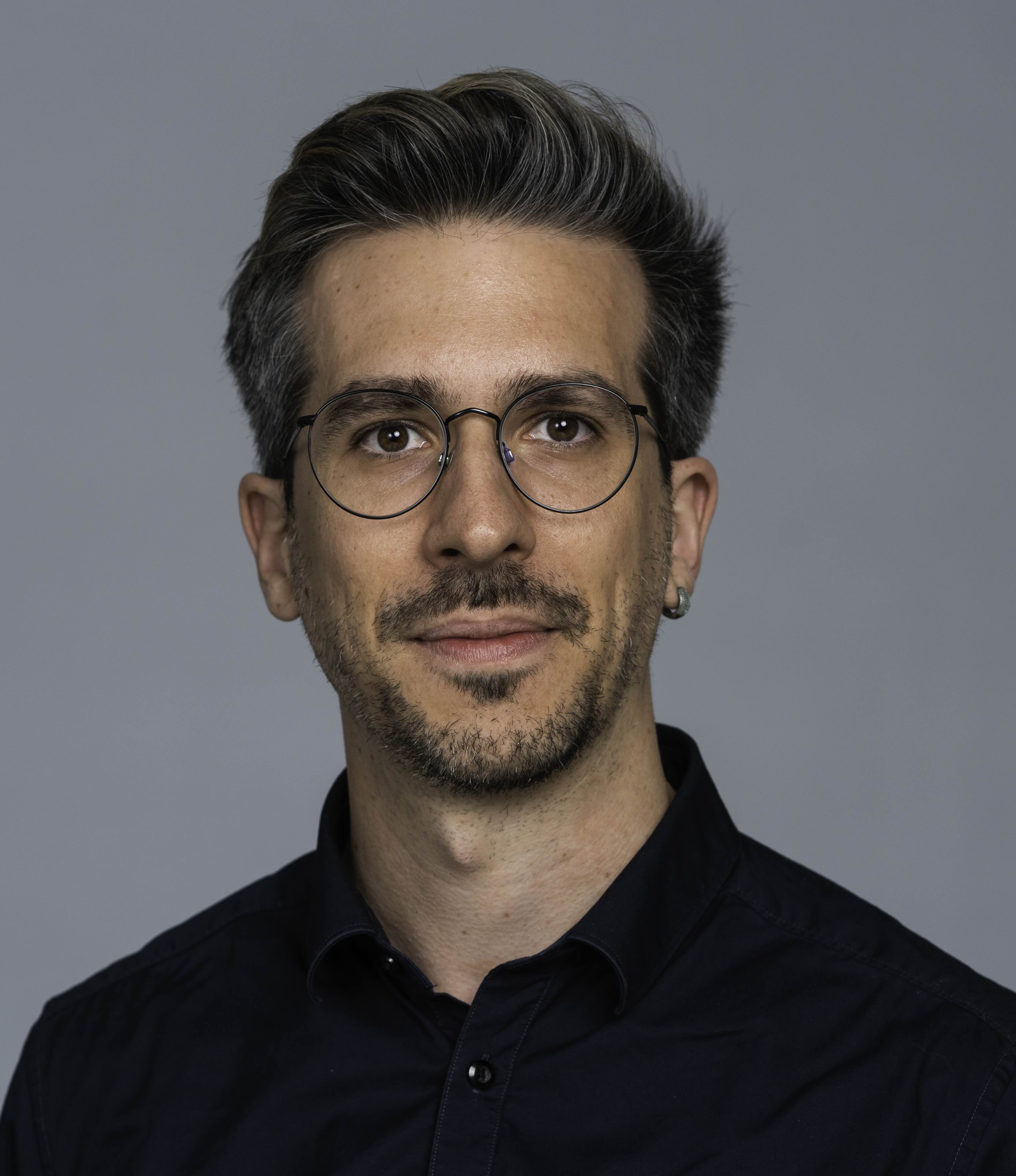
As a Lead Data Scientist at Novo Nordisk, I design and deploy AI/ML methodologies for early target discovery and precision medicine — with a strong focus on genetics.
I have 13+ years of experience in computational biology and applied statistics. I completed my PhD at CBIO, a leading bioML laboratory, under the supervision of Chloé-Agathe Azencott, where I explored graph-based methods for genetic studies. I then conducted my postdoctoral research at RIKEN AIP with Makoto Yamada, developing novel AI/ML methods for feature selection.
My research focuses on multi-omics integration, explainable ML and systems biology. I’m an advocate for reproducible research and an advanced Nextflow developer. I’m detail-oriented, proactive, and wired to fix what’s broken — ideally before anyone notices.
news
Apr 29, 2025 | Our preprint on deep learning-based genetic risk prediction is out! This work was driven by Taiyu Zhu from the University of Oxford. |
---|---|
Nov 15, 2024 | I was promoted to Lead Data Scientist. I will be working on AI/ML for target and biomarker discovery. |
Mar 07, 2024 | Our model for cardiovascular disease risk prediction got highlighted in Novo Nordisk’s Capital Markets Day. |
latest posts
May 29, 2025 | DNA language model fine-tuning and inference |
---|---|
Apr 25, 2025 | An intro to uv |
Apr 01, 2025 | SHAP values |
selected publications
- Interpretable Machine Learning Leverages Proteomics to Improve Cardiovascular Disease Risk Prediction and Biomarker IdentificationmedRxiv, 2024
- A network-guided protocol to discover susceptibility genes in genome-wide association studies using stability selectionSTAR protocols, 2023
- Interpretable network-guided epistasis detectionGigaScience, 2022
- Block HSIC Lasso: model-free biomarker detection for ultra-high dimensional dataBioinformatics, 2019
- The functional impact of alternative splicing in cancerCell reports, 2017